
This comprehensive guide will embark on an enlightening journey into the captivating world of generative artificial intelligence (AI). Generative AI, a cutting-edge field combining machine learning and creativity, has revolutionized how we approach various domains, from art and design to music and storytelling.
Throughout this guide, we will delve deep into generative AI’s fundamental principles and advanced techniques, empowering you with the knowledge and skills to unlock its incredible potential. Whether you are a beginner seeking an introduction to the subject or an experienced practitioner aiming to enhance your expertise, this guide will provide valuable insights and practical guidance.
We will explore the underlying algorithms and architectures that drive generative AI, such as generative adversarial networks (GANs), variational autoencoders (VAEs), and deep reinforcement learning. From understanding the intricacies of training models to generating realistic images, synthesizing novel music compositions, and creating virtual characters, you will discover the diverse applications and possibilities of generative AI across industries.
Moreover, we will examine the ethical implications and considerations associated with generative AI, emphasizing these powerful tools’ responsible and ethical use. As generative AI development services evolve and shape the future, it is crucial to grasp its societal impact and address potential challenges.
Whether you aspire to become a generative AI researcher, an artist exploring new creative frontiers, or a professional seeking to leverage generative AI in your field, this guide will equip you with the knowledge and resources needed to embark on your journey. Let us delve into the exciting realm of generative AI and unlock the door to limitless imagination and innovation.
Understanding Generative AI
Generative AI encompasses various techniques and models designed to generate new content. Autoregressive models form one category of generative models. These models predict the conditional probability distribution of data by examining the preceding tokens in a sequence. Language models like OpenAI’s GPT excel at generating coherent text by leveraging autoregressive techniques. Similarly, autoregressive models find applications in speech synthesis and other sequential data generation tasks.
Another significant class of generative models is Generative Adversarial Networks (GANs). GANs consist of two components: a generator and a discriminator. The generator generates new samples, such as images, while the discriminator distinguishes between the generated samples and real samples from the training dataset. Through an adversarial training process, the generator aims to create indistinguishable content from real data, while the discriminator continually improves its ability to differentiate between real and generated data. GANs have revolutionized generative AI, enabling image synthesis, style transfer, and video generation advancements. However, applying GANs in creating deep fake videos has raised ethical concerns, emphasizing the importance of responsible AI usage.
Variational Autoencoders (VAEs) represent another notable technique in generative AI. VAEs combine generative modeling and autoencoders, neural networks designed for data compression and reconstruction. By incorporating a probabilistic framework, VAEs can generate new data points while reconstructing the original input. VAEs find applications in image generation, anomaly detection, and data augmentation, where the synthetic data generated by the model enhances the performance and robustness of machine learning models.
Reinforcement Learning (RL) techniques can also be employed in generative AI to create models that learn to interact with their environment and generate rewards-based content. RL-based generative models have been used to generate game levels, compose music, and provide personalized recommendations. Using a reward system, these models learn to optimize their content generation process based on desired outcomes, showcasing their adaptability and versatility.
Also Read: Chatbot Development in 2023: An Ultimate Guide
Applications of Generative AI
Generative AI techniques have found widespread applications across various domains, transforming how we create and interact with content. Here are some notable examples:
- Image Synthesis: Generative models can generate realistic images, leading to applications in image editing, virtual world creation, and product prototyping. Artists and designers can leverage these models to bring their creative visions to life.
- Music Generation: AI models trained in generative music composition can create original compositions in various styles and genres. This technology opens new avenues for musicians and composers, inspiring them with novel melodies and harmonies.
- Text Generation: Language models, such as GPT, have been trained extensively to generate coherent and contextually relevant text. These models are valuable for applications like chatbots, content creation, and creative writing assistance.
- Data Augmentation: Generative models can generate synthetic data to augment training datasets, improving machine learning models’ performance and generalization capabilities. This approach is particularly useful when the availability of real data is limited.
- Virtual Reality and Gaming: Generative AI is vital in creating immersive virtual environments by generating realistic characters, landscapes, and interactive content. This enhances the gaming experience and opens new possibilities in virtual reality applications.
- Healthcare and Drug Discovery: The healthcare industry benefits from generative AI in various ways. It aids in medical imaging analysis, drug discovery, and personalized medicine. Generative models can assist in interpreting medical images, providing insights, and assisting healthcare professionals in accurately diagnosing diseases. In drug discovery, generative AI techniques can generate novel molecular structures and optimize drug candidates, accelerating the development of new therapies. Additionally, generative models can personalize treatment plans by analyzing patient data and generating tailored recommendations based on individual characteristics.
Challenges and Future Directions
While generative AI holds immense potential, several challenges must be addressed for further advancements. One of the primary challenges is ensuring the ethical and responsible use of generative models, particularly in areas such as deep fakes, where the potential for misuse and misinformation exists. Stricter regulations and guidelines are necessary to mitigate these risks and maintain the integrity of content generation.
Additionally, improving the interpretability and control of generative models is a critical area of research. Enhancing the transparency of the models’ decision-making processes and giving users more control over the generated output will contribute to their wider adoption and acceptance.
The future of generative AI looks promising, with several exciting directions for exploration. Researchers are actively working on enhancing the realism and diversity of generated content, enabling models to create content that is even more indistinguishable from human-generated content. Additionally, advancements in generative AI are expected to contribute to interdisciplinary fields, such as augmented reality, where realistic and interactive virtual environments can be seamlessly integrated with the real world.
Conclusion
Generative AI techniques have revolutionized content generation across various domains, showcasing their ability to create original and realistic content. Autoregressive models, GANs, VAEs, and RL-based approaches have opened up new possibilities in image synthesis, music composition, text generation, data augmentation, virtual reality, and healthcare. While challenges and ethical considerations persist, ongoing research and responsible use will shape the future of generative AI.
As the field continues to evolve, researchers, practitioners, and policymakers need to collaborate in establishing guidelines and frameworks that ensure the responsible and ethical deployment of generative AI. We can unlock unprecedented creativity and innovation in various industries by harnessing the power of generative AI techniques and applications, shaping a future where AI-driven content generation becomes integral to our lives.
You might also like our TUTEZONE section which contains exclusive tutorials on making your life simpler by using technology.
Author Bio:
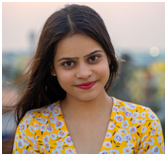
Aditi Shah is a seasoned digital marketer passionate about optimizing online presence and driving organic growth. With over two years of experience in SEO, social media marketing, and content curation, she has honed her skills in crafting compelling strategies that boost brand visibility and engagement.